In this episode of Ten Thousand Feet: The OST Podcast, we dive into the world of artificial intelligence (AI). We are joined by OST CIO and co-founder Jim VanderMey and enterprise cloud architect Brian Partin. We’ll define the term, discuss how clients are exploring the new technology, and cover the benefits and limitations of AI.
In the episode, Jim shares a few resources in this space — explore below:
- Capturing Users’ Reality: A Novel Approach to Generate Coherent Counterfactual Explanations
- Article: The Jobs Most Exposed to ChatGPT
- Book: The Age of AI by Henry Kissinger, Eric Schmidt and Daniel Huttenlocher
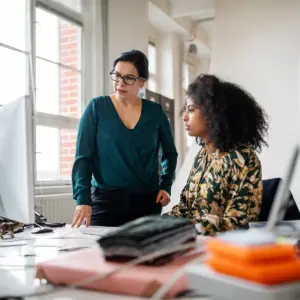
Generative AI: Learn and Build in a Sandbox Environment
This podcast content was created prior to our rebrand and may contain references to our previous name (OST) and brand elements. Although our brand has changed, the information shared continues to be relevant and valuable.
Episode Transcript
Kiran: Hello, welcome to today’s episode of Ten Thousand Feet: The OST Podcast. I’m your host, Kiran Patel. I’m joined today by OST CIO and co-founder Jim VanderMey and Enterprise Cloud Architect Brian Partin.
Jim, welcome to the show.
Jim: Thank you, Kiran.
Kiran: And Brian, it’s great to have you.
View Full Transcript
Brian: Good morning, Kiran. Happy to be here.
Kiran: Awesome. So in today’s episode, we are going to dive into one of the hottest topics in the news right now, and that is AI. You’ve heard about it, you’ve read about it, perhaps you’ve even experimented with it a little bit on your own. And we want to bring some of our experts into the conversation and see what’s on the horizon, in the forefront for us here at OST.
So if we’re going to talk about AI, we should probably get on the same page about the definition of this term. So let’s begin by defining it.
Jim, when you hear artificial intelligence, what does this term mean to you?
Jim: Right now, AI is being used for a lot of different capabilities, but I tend to lean towards, it’s a collection of trained models. It could be a neural network, it could be machine learning, it could be a deep learning model that actually takes actions based on the predictions, not just providing insights or recommendations. So it eliminates the need for human intervention.
Ideally, it would get smarter over time, and so there are places where AI can discover patterns that humans had here before, had never been able to see as it looks at the data sets that we supply them. And that idea that it’s a neural network, machine learning, deep learning that is able to drive prediction and also learns from additional data as it becomes available.
Kiran: Excellent. Brian, what would you add to that definition?
Brian: So first, I just want to point out that what Jim was walking through is super important. If you look at the more traditional definition, it would’ve included things that I think now, we just think of as part and parcel of computing. The ability to look at a large amount of things, throw out an answer based on that assessment without any of that ability to learn, ability to see patterns that aren’t just pure probabilistic math.
I do think that we’ve arrived at a point where it’s a much more honed definition that we’re all working with. And I think part of that is because what’s causing the buzz now is generative AIs. AIs that display human-like characteristics in terms of being able to do quasi-creative activities, I think that’s what’s causing the buzz as opposed to some of the earlier models.
Kiran: Absolutely. Brian, I want to ask you, in your space, in your work in the enterprise cloud space, I’m certain you’re consulting with clients who are encountering similar conversations in the generative AI space.
What are some of the concerns you’re fielding and you’re hearing from clients, and what are they experimenting with some of these new iterations?
Brian: So it’s interesting right now because we’re at that pre — we’re still approaching a definition of where AI really is culturally. I’m not, right now, hearing people ask about what should I be concerned about? I’m hearing more, I wanna run with this right now. So, there’s a tendency to have to pull back on the reins a little bit, get them to think about what they’re trying to do, and realize that it isn’t a magic button.
I think it was Arthur C. Clarke who said, “Any sufficiently advanced technology is indistinguishable from magic.” I think what we’re seeing now is magical thinking in this space with businesses. This is like any other technology. You have to slow down, look at your use cases. Do the definition like we did at the kickoff. Find out where that definition applies and then understand what you’re getting into.
Kiran: Jim, I think you were sharing some of these anecdotes as we were preparing for the conversation, but this, in fact, is not new. We’ve had versions of this around for a while.
What do you think is causing some of the buzz right now, or just generating a lot of the excitement that we’re hearing now?
Jim: I think that clearly, ChatGPT 4 and some of the OpenAI models is driving that excitement. And I think that one of the aspects of any new technology is, how testable is it? How trialable is it? And we’ve now hit a point where, in the popular spaces, people can go out and try something that until, very recently, required access to data science tools and specialized equipment knowledge and models, and it’s really been democratized at this point so people can try it out and test it.
And so I think that testability, it’s like when the PC first came out in the 1980s, the idea that you could have a computer on your desktop was a very democratizing moment for technology. I think that this is that moment for AI.
Kiran: Brian, have you heard from people and perhaps even yourself, as you’ve been experimenting with some of these generative technologies?
What’s been your take on it? Has it been a positive experience for you, or what have you enjoyed about it?
Brian: So I’ve been playing one way or another in the AI space since the mid-90s when I was in banking and we were using it then. Again, back then, it was the more primitive version of AI, the one that Jim was careful to distinguished from what we’re seeing today. But the difference between now and then is what Jim was getting at. It’s far easier to experiment, to run things through its paces. I’ve been playing with — the second these models were released, I started working with them. I started incorporating them into work I’m doing with my clients.
The fact that I can test, I can retest, and I get results that are in line with what I would expect in terms of testable responses, similar answers that I would get if I were to empanel a group of individuals with some expertise in a topic. So it’s a marvelous research tool. It’s a marvelous way to get access to a large amount of information, condense it down so that you can make appropriate choices. Even when it comes down to doing things like imagery for presentations. I’ve been testing the bounds with some of the generative capabilities in terms of imagery. Put in an idea, see what it comes up with.
That’s been kind of, that whole creative aspect has been the thing that’s been missing, and that’s, I think, the part that’s very exciting. The Turing test part of it, conversing with ChatGPT. Yeah, that’s awesome. It’s amazing.
But the stuff that to me is the most different than from what I’ve seen in the past, is what’s going on right now with quasi-creative pursuits. It’s not perfect. But then again, human actors don’t produce perfection on the first go around.
Kiran: Sure. Jim, how about yourself? Have you had a chance to experiment in a few different ways? I’m wondering about your own interactions with some of these generative platforms and what your takeaway has been.
Jim: I think it’s very similar to what Brian said, is that using it as a tool to to assist. So the idea that I might ask a question or pose a scenario or even create an image, that it allows me to effectively have a ChatGPT thought partner for things that maybe I wouldn’t have thought about. Maybe nuances that I wouldn’t have thought, maybe variables I wouldn’t have considered in the discussion. And so there’s that piece of it.
I was involved in a project a few years ago where we took models that were developed in one institution and we then took them into another institution to see if the predictive modeling that would identify a certain condition in the patient would be something that we could generalize from one institution and data set to another institution and data set. We found out that it actually didn’t translate. And so the methods translated, but the models didn’t directly translate.
And I think that’s something similar to what I’m seeing now, is that our methods of engaging with AI remain consistent and we can continually use these methods over and over again, but the actual output from them is typically very narrowly applicable to a specific problem, a statement, or a data set. We don’t have generalizable AI yet.
Brian: What’s fascinating about what Jim’s talking about is the rise over the last six, seven months, in a new job class within IT, the prompt writer. The person who knows how to go in and ask the right questions.
It’s interesting because a lot of what I do as an enterprise architect is go in and question humans. Try to find out, listen to their responses, try to figure out what their meaning is, see if their meaning shifts when I move from this person to another, and it’s really interesting to me that we’re at the point with generative AI that we can start working that same game. But it’s that whole refining, that need to get down to it, these are not a plug-in replacement for human decision making. What they are is, it’s like a pry bar as an extension of your arm in the same way — or that a computer, what makes IT so attractive is, I don’t have enough memory to remember every single client that we’ve had through all time. I can reach out and use a database as an extension of my mind. ChatGPT is just a way to temporarily shrink my ability to get to salient information.
Jim: In March 28, the Wall Street Journal had an excellent article about jobs that are going to be changed through the use of GPTs, and what they were describing is that there are some jobs that are going to be virtually unaffected. Short order cooks, motorcycle mechanics, and oil and gas roustabouts were at the top of the list of jobs that were not going to be affected.
But Kiran, your job was at the top of the list. Public relations specialists, court reporters, blockchain engineers. People who are knowledge translators and classifiers are highly exposed. And this particular study that was referenced, it found that 80% of workers in those types of occupations are going to have at least one job task that can be performed more quickly by using generative AI.
Brian: It’s interesting. The other, another category that I haven’t seen a lot of discussion on, but is certainly also going to be affected, is that whole layer in large organizations that sits just below the executive branch, where they’re effectively acting as collators and aggregators of information as part of the decision process that happens strategically within an organization. It’ll be interesting to see how much that class is effective because their job is effectively doing what ChatGPT does and similar constructs do, which is gathering a huge amount of information. Looking at it within the context of the model they’ve been given, if that model’s a business model, it seems to me executive leadership would want to take advantage of that rapidly. So it’s gonna be interesting seeing where this hits. It’s gonna be very different than the Industrial Revolution. And in many ways, the beginning of computerization.
Automation’s affecting very different places in our culture than it has in the past.
Jim: Brian, that particular example of the collation of information within an enterprise with — and we’re focusing a lot on generative AI right now — the ChatGPT model, because that’s what’s got all the buzz. But that layer of the organization that is right below the executives, as you mentioned, one of the problems that AI has is its opacity, and that is that you don’t know why that particular insight was elevated above other insights. The ability to drill in and understand. Because imagine you have a director in an organization who has done that collation, they bring a conclusion forward and then you ask, why did you have that conclusion? They can answer that question. They can go back to source data and show that. ChatGPT can’t do that.
Brian: Yeah, and it’s one of the things that’s being pointed at right now from the technical standpoint. If you look at the academic literature, it’s more looking at what’s the next step in AI. It comes down to determining how to be able to do that, get that observability within it so you’ve got access to the data. Because in the end, what ChatGPT, what you want it to do is pull out that information together, understand where it came from, and then get its recommendations.
The part that’s interesting about it is that then eliminates some of the concerns that you have. In theory, when you have human actors collating information, and there’s an opacity with why they came to a certain conclusion. Might be better for them than the organization, might be better than this. So there’s this really interesting ethical, moral, and procedural problem playing out in some of the research papers I’ve seen within the last couple of weeks. It’s really fascinating, and again, because it’s happening at that top of organizations, it’s particularly interesting.
Kiran: Certainly. I think what I’m gleaning is, while the technology is exciting and worth experimenting with, it’s wiser to see it as a complement to what we do rather than a replacement of what we do.
And I’d advocate that there are just certain things that it can’t yet do, such as convey emotion, although I know that these technologies are constantly learning. So I see it as again, a tool to use that I can have in my toolkit rather than it using me, or so on and so forth.
But Brian, you mentioned the ethics question, so I wanted to dive in a little bit to some of the ethical obligations that come up as we talk about such technologies. What are you seeing in that space?
Brian: I think if we were to break it down into kind of the top five things, ethically, morally, to look at that we have to consider with it, it’s gonna come down to fairness, privacy — the concern of everyone right now, security, transparency, explainability — which is related to that transparency that Jim was getting at, and then accountability. All of those things, in the same way that human actors are responsible — organizations are responsible for the human actors, following those same five things, I think we have to treat AI in that same way if we’re going to trust it to do tasks where a human would be held accountable to certain things, so too does the AI. We have to have the same ability, if not arguably better ability to drill in and understand where things are at, and ensure that those things are meeting the baselines.
Kiran: Is there anything you wanted to add, Jim, in that space? As far as ethical obligations?
Jim: There are a huge number of ethical obligations, and some of which I would say are, as Brian said, is to think about the data and the biases in the data that inform the training then of that. And in the materials with the podcast, I’ll have a couple of books that I would recommend in that space.
And then the other piece of the ethical obligation then translates into, I’m gonna call the legal frameworks. You’re seeing this right now in the autonomous vehicle spaces, where if you have a self-driving car or you have a brand promise that for automobile braking systems, for example, and that doesn’t detect a critical event.
There was a great example a few years ago in 2017 when Volvo brought their autonomous vehicles to Australia. Their large animal detection algorithms could identify caribou and moose and deer, had no idea what a kangaroo was. And if someone had an accident with a kangaroo with an autonomous vehicle, and they’re sitting in the driver’s seat, whose responsibility is that? Is it the algorithm developer who didn’t have the right data set? Is it the driver? And right now, we say that the driver, the owner of the vehicle is where culpability lies. But as algorithms take over more work in that assistive capacity — so for example, if you’re using a GPT assistant and you create something that is slanderous or libelous and you use that, are you gonna blame the AI because it supplied something to you that had strong bias associated with it?
And so I think that how we use these tools, cognizant of what the deficiencies are, is very important. And also, making it clear to companies’ customers, making it clear to a person, that they’re not actually interacting with a human being in this moment. That you’re interacting with a chatbot. Because in organizations’ desire to scale their call centers, to engage patients, to drive costs out of an organization, they could very easily create things that are looking or acting like humans in a limited way, but you’re actually interacting with a chatbot. And I think that it’s important to be honest with people about that.
Brian: I was just in the background shaking my head so much, I think the rocks inside were rattling around. But it is that whole — our whole legal system, our way of adjudicating risk, accepting risk, sharing risk across human interactions. It all gets tipped up and poured out on the ground the minute AI steps into it. We look at, as an example from a legal framework standpoint, whose fault is it that the kangaroo got hit? You come back to it though and you look at it, AI per unit model driven is gonna have far fewer accidents than humans.
So there’s an actuarial argument that happens that is wrong — my opinion, from an ethical standpoint, it’s wrong — but it’s often applied in support of just allowing AI to continue without thinking about that transparency, thinking about those controls, and holding the folks accountable who train and continue to train the models. One of the big concerns I would have is any AI model that goes out there, each of the — whether it’s discrete agents that are out on the edge, whether it’s all working remotely and connecting back to a master AI. All of that has to be constantly learning, adapting, but all of the ethical, moral, and model obligations, all that training has to continue to happen, to keep it within the guardrails that were set up or that may change over time.
And it’s not just fire-and-forget. You don’t throw an AI out there and leave it. It won’t work.
Kiran: Jim, could you share the framework that you use when you help our clients think through some of these technologies? Because I believe it’s applicable here when we talk about AI, and it speaks to how we’re positioned to help our clients think through this space.
Jim: Sure, Kiran. So we’ve been working in spaces where technology and human experience intersect for a long time, and our approach is that whenever you’re creating a novel technology solution that inevitably, people need to enter that system and they need to exit from that system with some data and conclusion. And some people will opt in, and some people will opt out, and some people will be inside of a system that are using AI and some people will be acted upon by that AI.
And so how you experience, or the first thing that we focus on, is what experience are you creating for the user? That’s a human-centered design conversation. It’s a service design conversation. And that understanding, that experience, is how we then drive those entry points, those exit points. And an example of that is in a healthcare chatbot application, for example, that if you are interacting with a chatbot in a virtual telehealth application and going through a triage mechanism, when you exit and are sent to your doctor’s office for scheduling, you would hope that all the information that you supplied to that chatbot would be consistent with the triage mechanisms that the doctor’s office would use and would be communicated to the doctor’s office so you don’t have to repeat yourself. So that shows how we move from the experience with the technology into the experiences for the users’ end both inside and outside the system. So that’s the first piece.
Does the platform you’re building allow you to ingest the data and analyze it at scale? So that’s a data engineering problem. We have to make sure that we’re building with the right data sets and on the right platforms. Is the content output or recommendations integrated into the workflow in order to engender trust?
And that’s where the explainable AI piece comes in to bear. And we see this with predictive maintenance algorithms because as soon as you present an expert with a counterfactual recommendation, a counterintuitive recommendation, the first thing they’re gonna do is question it. So you have to have ways to make sure that you’re engendering trust in the user of the product, that you’re not creating something that either creates nonsensical responses because you’re gonna decrease trust, or opaque responses that decrease trust.
And the fourth area is that it’s not just about collecting the data. It’s knowing how it’s going to be used and identifying the behavioral impact that you’re trying to drive through this. The human behaviors and the outcomes from that are part of the design.
So what experience are you trying to create? What is your platform, architectures, and engineering? How are you integrating into existing workflows, and how are you engendering trust? And then having that understanding of how we’re going to use and what behaviors and outcomes we’re trying to drive towards.
Kiran: I know those are themes that have come up in the past and have remained consistent as we approach. I’m certain they’ll be relevant in the future too.
Just, Brian, from you, any thoughts or words of wisdom to those who are excited and eager in this space and just wanna run ahead full steam — just anything you might say to them? So they’re thinking about this technology in a way that could be advantageous.
Brian: It’s — curb your enthusiasm. None of this is a magic button. It’s like any other technology. It’s like any other tool. You have to understand your own business, what your goals are, what your goals are not just right now, what your goals are in the future. You have to go in and understand which of the processes, procedures, workflows within your organization where AI is applicable.
You then have to find the right model. There’s not a single AI model that’s usable for everything, so you have to find the right one. You have to determine whether you’re going to keep it off the rack, or whether you’re going to invest and create a whole new job type within your organization for maintaining that model, adding to that model, or whether you’re gonna stick with generic one.
There’s a lot to this. It’s like any other technology. It’s like when containers first came out, everybody flocked to it. A lot of people ran away. Or when cloud first came out, they flocked to it. They spent a lot of money, and they tried to operate in the way they had on their previous platform. Doesn’t work like that. You’re trying something new. Take your time. Understand it. Figure out where you fit. Don’t overstep. Be disciplined. I guess that’s the best answer, is be disciplined. Watch where you’re putting your feet.
Kiran: Certainly. There’s no silver bullet, of course. You have to —
Brian: Of course not.
Kiran: Be wise, be thoughtful, be intentional, ask a lot of the key questions in order to attempt to find success.
Brian: There’s a term in innovation called the peak of inflated expectations. You wanna wait until you’re past that. If others wanna rush forward while something’s still immature and they wanna risk it, learn from their mistakes.
Innovation by itself, whether it’s innovating by using AI or any other innovation, the odds you’re gonna be right and get success are very low. Take small bets, like any other technology. Take small bets. Don’t tie 25 things together that have to happen that all have low odds, or it’s not gonna work. So be discreet in how you approach things, and learn as you go, and adapt. The same thing we want out of a good AI system. The ability to view the room, see what’s happening, and adapt. You need that same thing in your own business processes. You have to be reflexive, self-reflexive.
Jim: I think there’s something though, that you just were talking about being — to avoiding entanglements, Brian. But I think that there is a necessary entanglement here, it’s that thinking that an AI innovation by itself will solve a problem.
We see many organizations that continue to struggle with using data effectively. And designing a dashboard for a fairly straightforward capability, understanding the right questions to ask, partnering with IT and the business for traditional analytics capabilities, trying to leapfrog that using AI without having strong partnerships between business stakeholders and IT stakeholders could just be the next toy in the toy box that sits on the bottom of the toy box and nobody actually plays with. And I think that it’s important to think that I’m solving a real problem with this.
And so right now, we’re at the stage of the POC to play with it and try things out. And it’s generating a lot of buzz, it’s getting a lot of hype, but understand the real human problem you’re trying to solve. And if you can link a real human problem to the technology, you can drive change in an organization, but it requires mutually reinforcing, simultaneous change in both the people and systems and the technology side in order for that to work. We used to call people, process, and technology. That’s a very real combination for this space we’re in right now.
Brian: Yeah, and just highlighting what Jim said, AI runs off the data. If you aren’t mature in your data space, there’s no AI that’s gonna be able to help you. You have to have a certain level of maturity before you step off into AI.
Jim: Well, AI is an accelerant. If you have bad data practices and —
Brian: You spread the fire.
Jim: Give you bad answers faster.
Kiran: Fascinating. We are gonna leave it at that for now. This is certainly going to continue to unfold as a conversation.
Jim, thank you for sharing your thoughts with us on the show today.
Jim: Thank you, Kiran.
Kiran: And Brian, great to have you.
Brian: Thank you so much, Kiran. This was an absolute blast.
Kiran: You’ve been listening to Ten Thousand Feet: The OST Podcast. OST, changing how the world connects together.